Updated: Feb 2021; the previous version (Mar 2017) of the research page can be found here
Our mission:
How does the brain represent, process, and regulate pain and affective experiences? The mission of our lab is to answer this question to better understand pain and emotional distress, thereby promoting the physical and psychological well-being of our family members, our friends, and our neighbors who suffer from pain and emotional distress.
Research topic list (links):
- Decoding brain representations of affect
- Psycho-socio-physiological modulation of pain
- Understanding natural thoughts, emotions, and pain through natural language and the brain
- Visceral AI
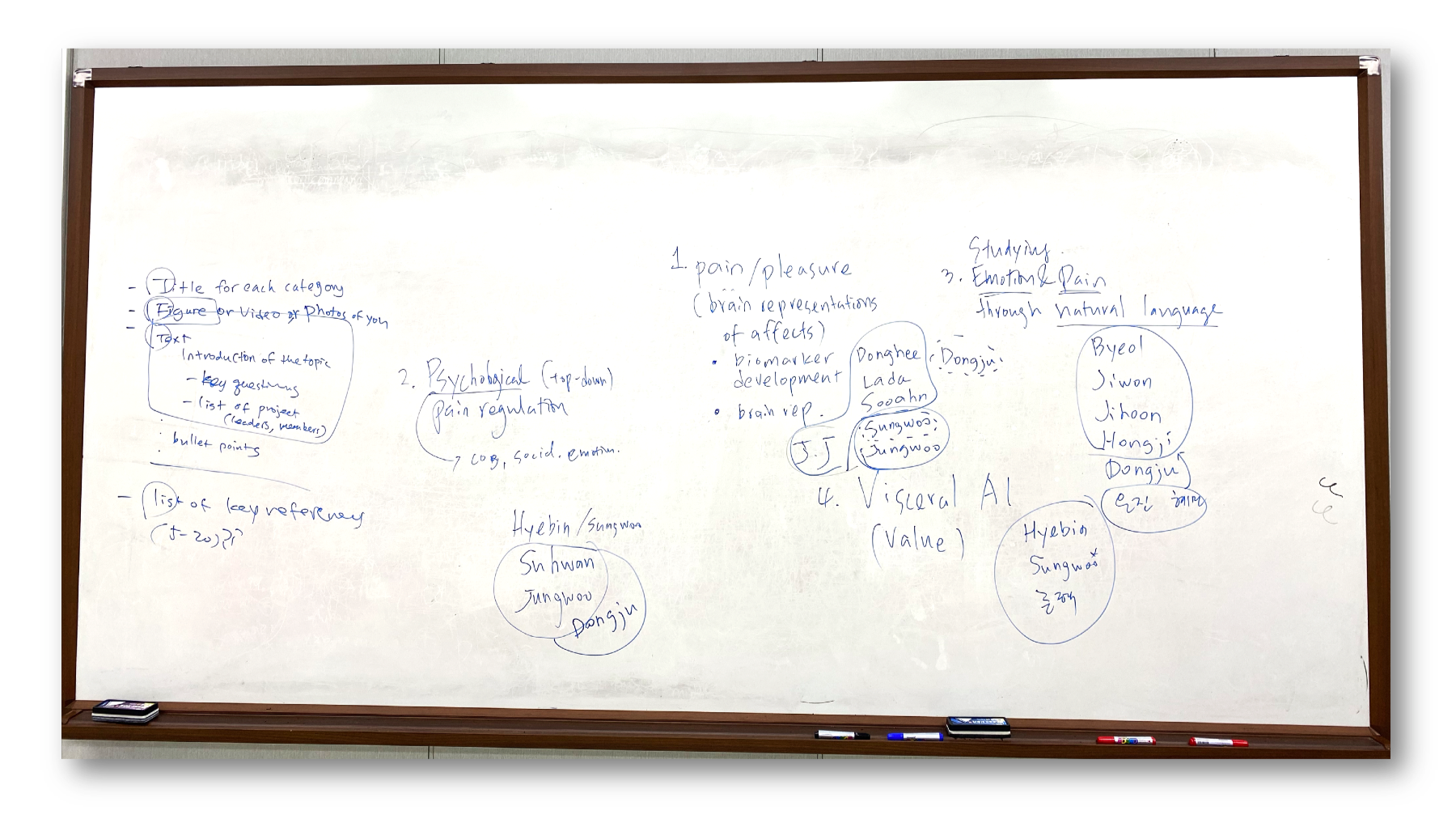
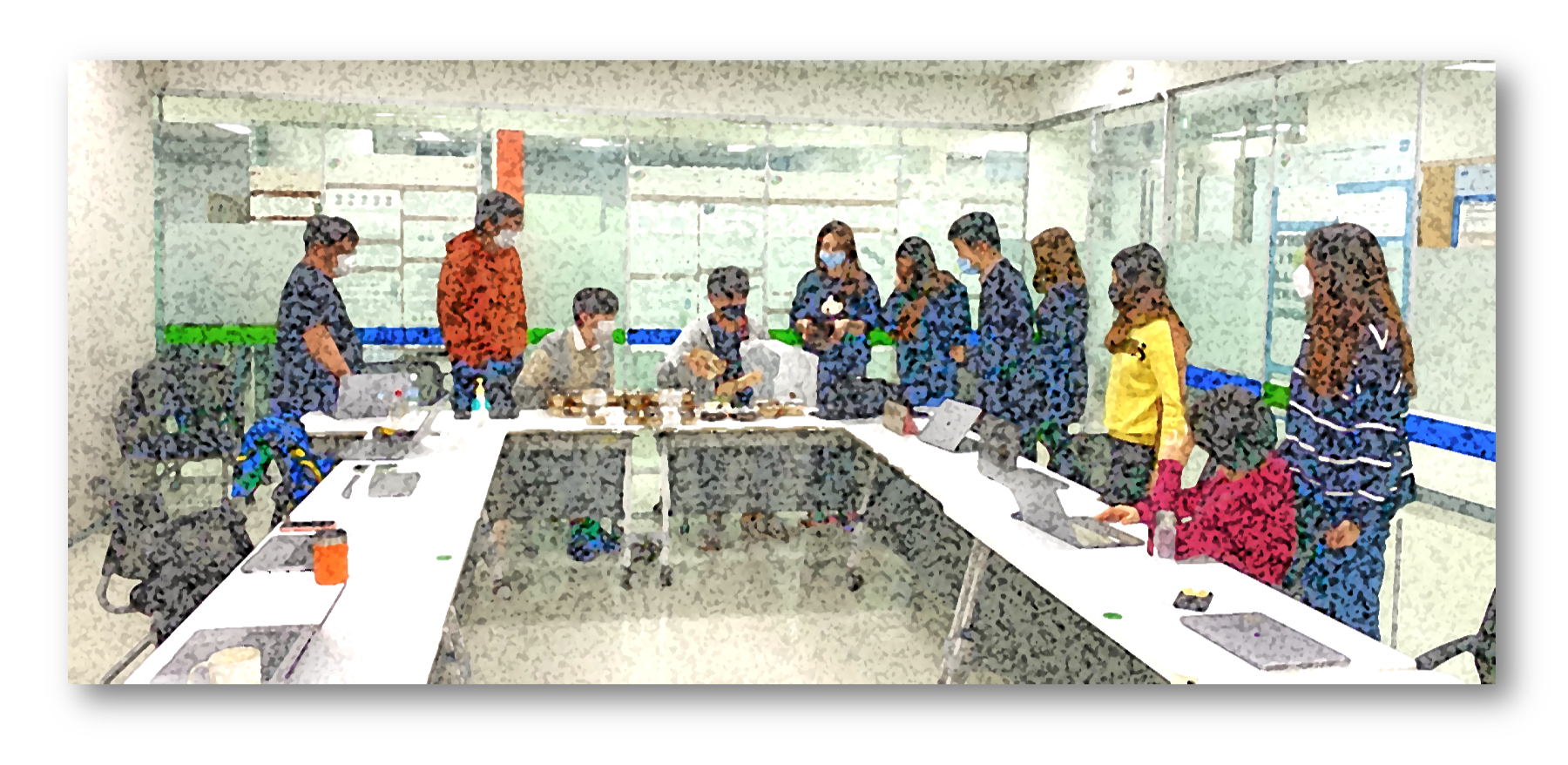
Decoding brain representations of affect
Pain is “an unpleasant sensory and emotional experience associated with, or resembling that associated with, actual or potential tissue damage” The revised definition of pain, IASP, 2020
How does the brain represent complex subjective and affective experiences, such as pain and emotions? Our lab aims to understand neural mechanisms of affective experiences with sophisticated techniques of fMRI and computational modeling. Particularly, we are focusing on developing “predictive models”, or “biomarkers”, of affective processes by modeling information distributed across the whole brain rather than relying on the traditional mapping approach (Fig. 1a). This predictive modeling approach not only generates testable hypotheses, but it also can provide a potential proxy of an affective process that can be used in clinical practices. However, there exist a number of methodological obstacles and challenges in the neuroimaging-based biomarker development. We strive to develop neuroimaging biomarkers that are reliable, trustworthy and useful, and generalizable across various contexts by using the systematic biomarker development and interpretation frameworks (Fig. 1b).
Key questions:
- How can we objectively measure and predict pain that an individual is feeling?
- How do the brain representations of pain differ across people?
- What is the relationship between pain and pleasure in the perspective of the brain?
- Developing brain biomarkers of cognitive and affetive styles for depression and chronic pain
Relevant projects (brief project description; leader; publication if any)
- XAIN (explanable AI in neuroimaging; Lada; Kohoutová et al., 2020, Nat Protoc)
- MBio (individual variability of pain representations in the brain; Lada, Donghee, & Sungwoo in collaboration with Tor Wager)
- LPP (the landspace of pain prediction; Donghee)
- MPC (mid-night pain club; highly-sampled fMRI data for pain; Donghee & Sungwoo)
- CAPS and CAPS2 (brain representations of sustained pain; Jaejoong; Lee et al., 2021, Nat Med)
- Pleasure (pain and pleasure; Soo Ahn)
- BMRK-rest (Rumination biomarker; Jungwoo in collaboration with Jessica Andrews-Hanna)
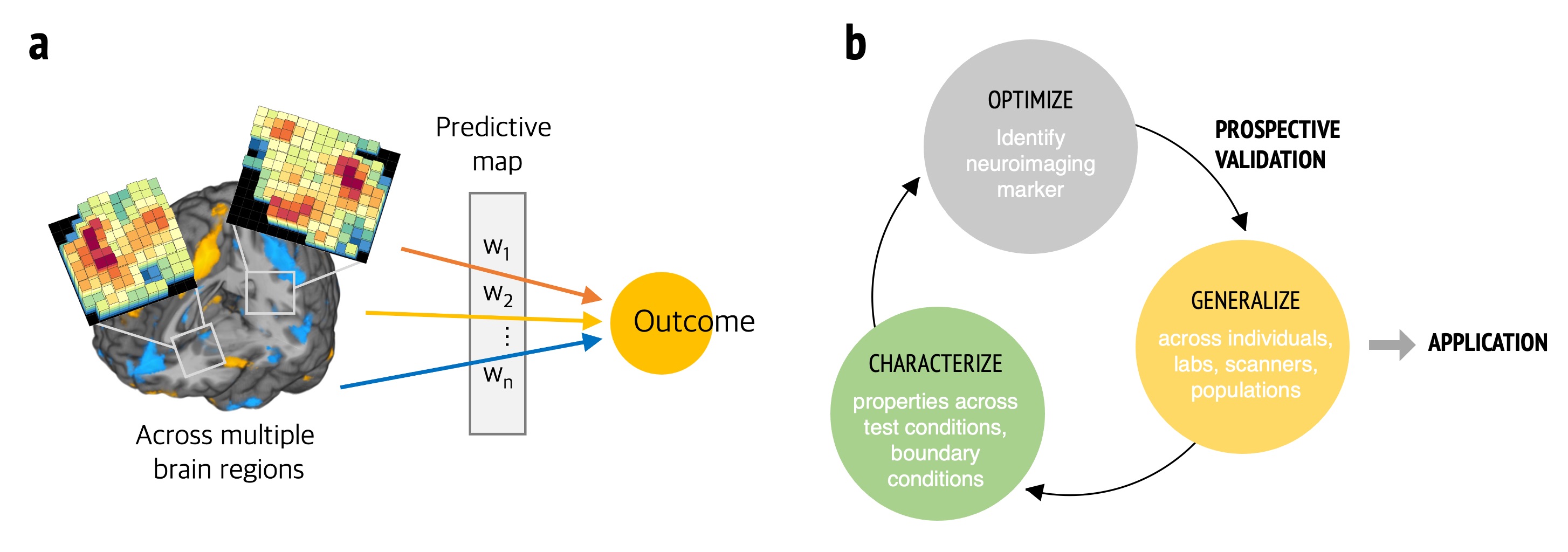
Psycho-socio-physiological modulation of pain
Unlike the old, but classical, conception of ‘pain,’ first proposed in 17th century by Descartes, researchers who now have advanced techniques to measure human brain functions and behavioral outcomes taught us that multiple factors beyond sensory inputs, e.g., social, affective, cognitive processes, substantially influence our pain experience. However, how those multi-factors can influence and construct our pain experience remains elusive. Our lab aims to understand what are the factors that contribute to our pain experience and their neural mechanisms using 3T and 7T MRIs (Fig. 2).
Relevant projects
- SEMIC (temporal dynamics of pain prediction using a semi-circular rating scale; Suhwan)
- SEP (social expression of pain; Suhwan in collaboration with Liz Losin)
- Pre-state pain decoding (Hyebin and Sungwoo)
- LSD_pain (layer specific decoding of pain; Jungwoo)
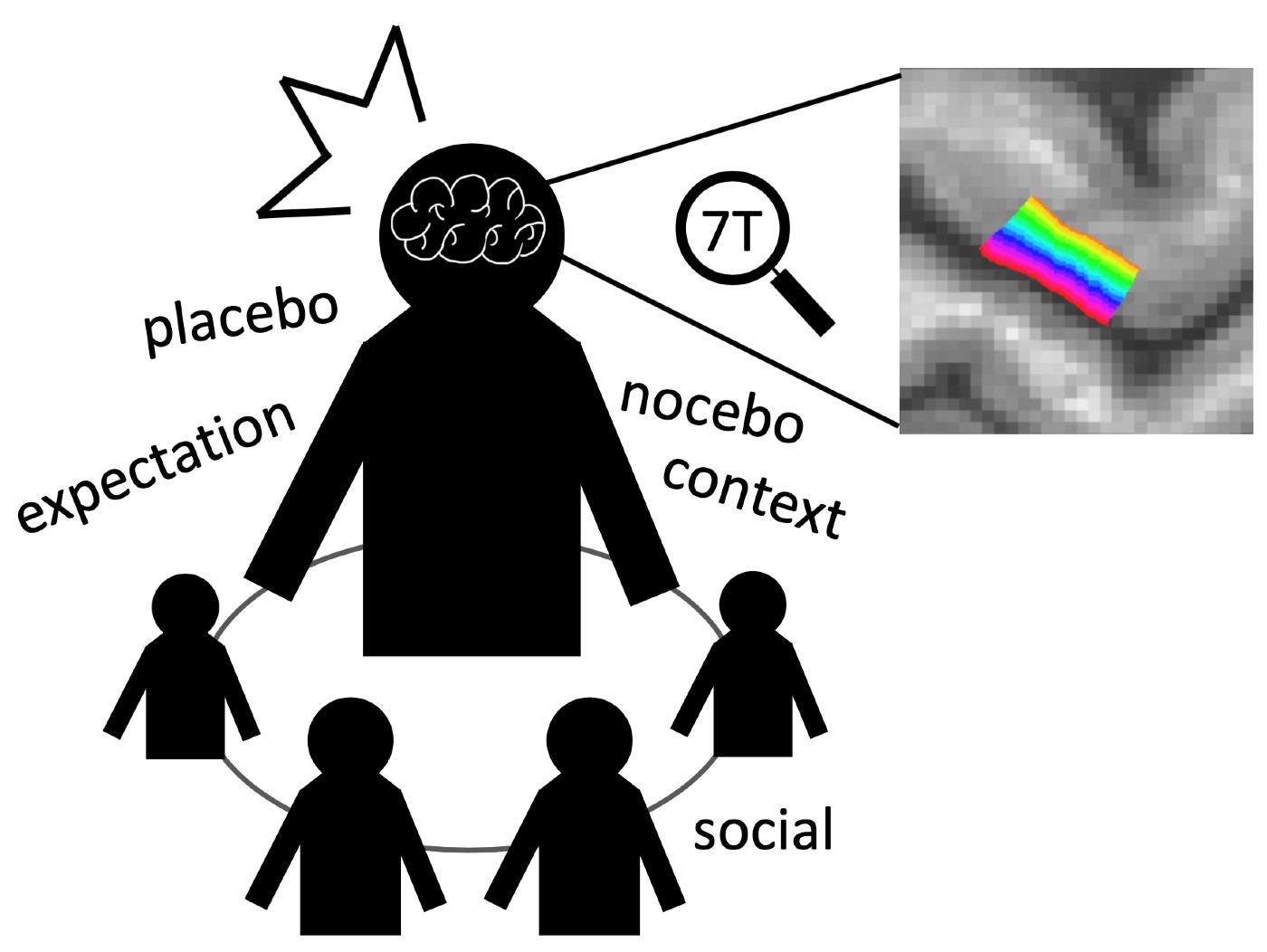
Understanding natural thoughts, emotions, and pain through natural language and the brain
We have been designing new naturalistic tasks to develop neural and behavioral decoding models for natural, endogenous thoughts, emotions, and pain. All these cognitive and affective processes are highly complicated experiences that are heavily influenced by internal and external contexts, past experiences, memories, expectations, and appraisal. We are trying to understand these subjective experiences using brain and language embeddings. We have conducted multiple fMRI and behavioral studies to develop neural and semantic bases of individual’s spontaneous thoughts and emotions and their dynamics (Fig. 3). Through multiple tasks, we tried to answer the following questions:
- Can we decode the affective dimensions of spontaneous thoughts by reading the brain activity?
- How are self-generated thoughts and emotions are processed and represented in the brain?
- Can we understand the semantic codes of the brain and how they are influenced by self?
Relevant projects
- FAST (free association semantic task; Byeol, Jihoon, and Eunjin in collaboration with Jessica Andrews-Hanna)
- FAST_nlp_network (Understanding the spontaneous thought with natural language processing and network modeling; Jihoon, Byeol, Eunjin)
- SiCo (Self in context with NLP; Hongji, Jiwon, and Jihoon)
- Spontaneous thought sampling (Hongji in collaboration with Emily Finn)
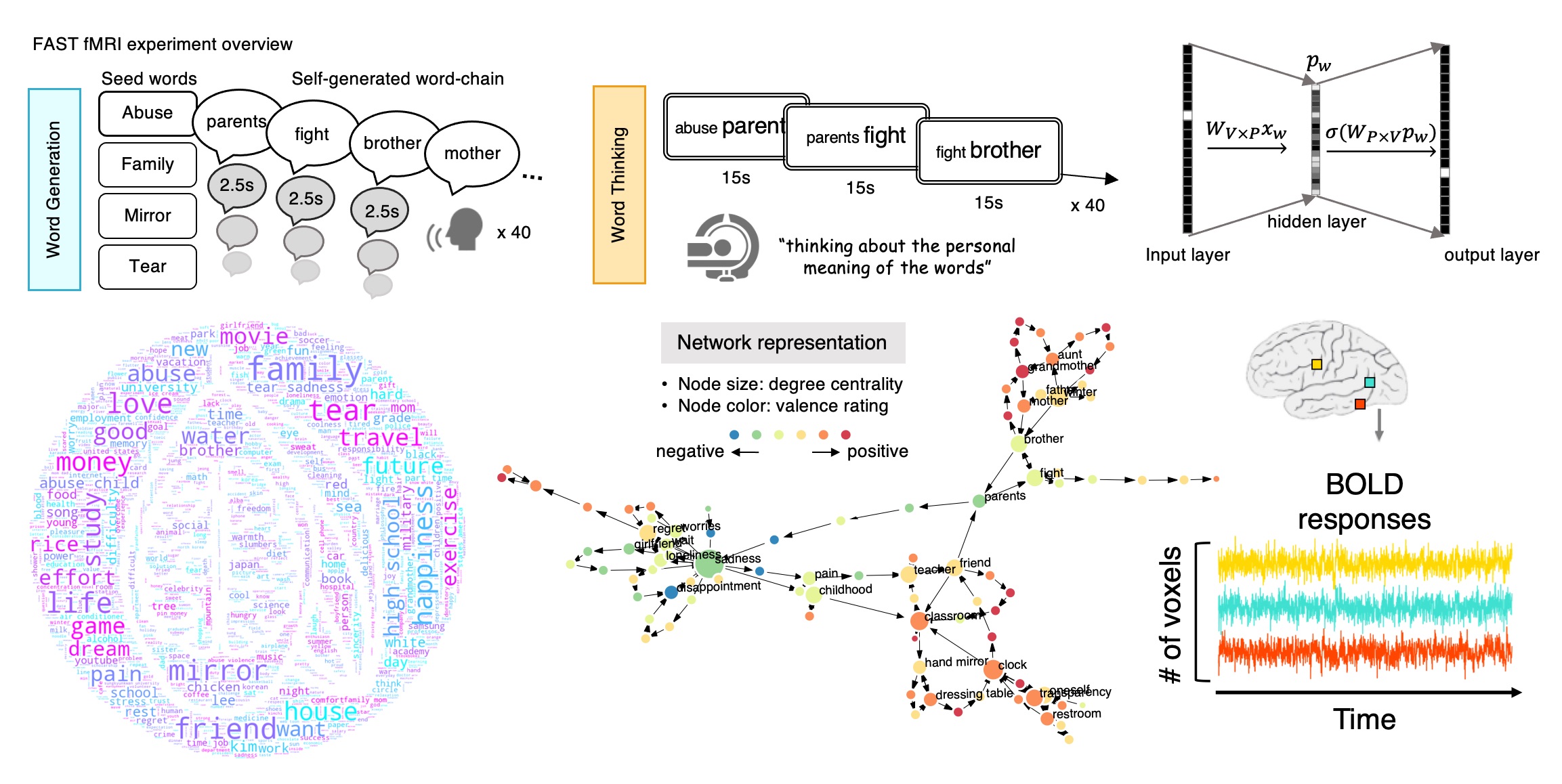
Visceral AI
“Rewards and punishments are sensory observations (the taste of an apple or the warmth of an embrace) and so stimulus value must be inferred by the agent, not conferred by the world. In other words, rewards must be intrinsic, not extrinsic.” - Juechems et al., 2019
There has been an impressive advance in artificial intelligence (AI), e.g., deep learning, which has attracted many neuroscientists. For example, vision and audition researchers found that the reprsentations of convolutional neural network can be mapped onto the brain (Yamins, 2014). This research has been focused on supervised learning tasks like image and sound classification. In this supervised learning framework, AI has no interactions with the environment. In the real world scenario, animals and humans not only passively receive information, but also influence the environment to change their state. Reinforcement learning (RL) can be an alternative approach to AI. Same as other AI fields, RL also made impressive development (Botvinick, 2020). This new approach called deep RL can offer a comprehensive framework to neuroscientists to study their hypotheses in decision making, value processing, etc. (Dabney 2020, Cross et al. 2020). However, even if we follow a deep RL framework, there are problems related to sparse reward problems and complicated reward designing. Also, there are some critics about value in reinforcement learning (Juechems et al., 2019). In order to handle these problems and understand animals and humans value systems, we have focused on intrinsic and internal value in AI (Fig. 4). This question is related to where value comes from in the real animals and humans. Animals track current and desired internal states and seek to minimise the distance to a goal across multiple value dimensions (Keramati, 2014).
Keywords and key questions
- Value: Where does value come from?
- Intrinsic value: What is the AI’s goal? Whose goal?
- Self, Homeostasis: What is the self in AI?
- Interception, Motivation: How are emotional states represented in AI?
Relevant projects
- Interoceptive AI (Sungwoo, Hyebin, and Junhyeok)
- Intrinsic Value Research Labs (a grant proposal is under review)
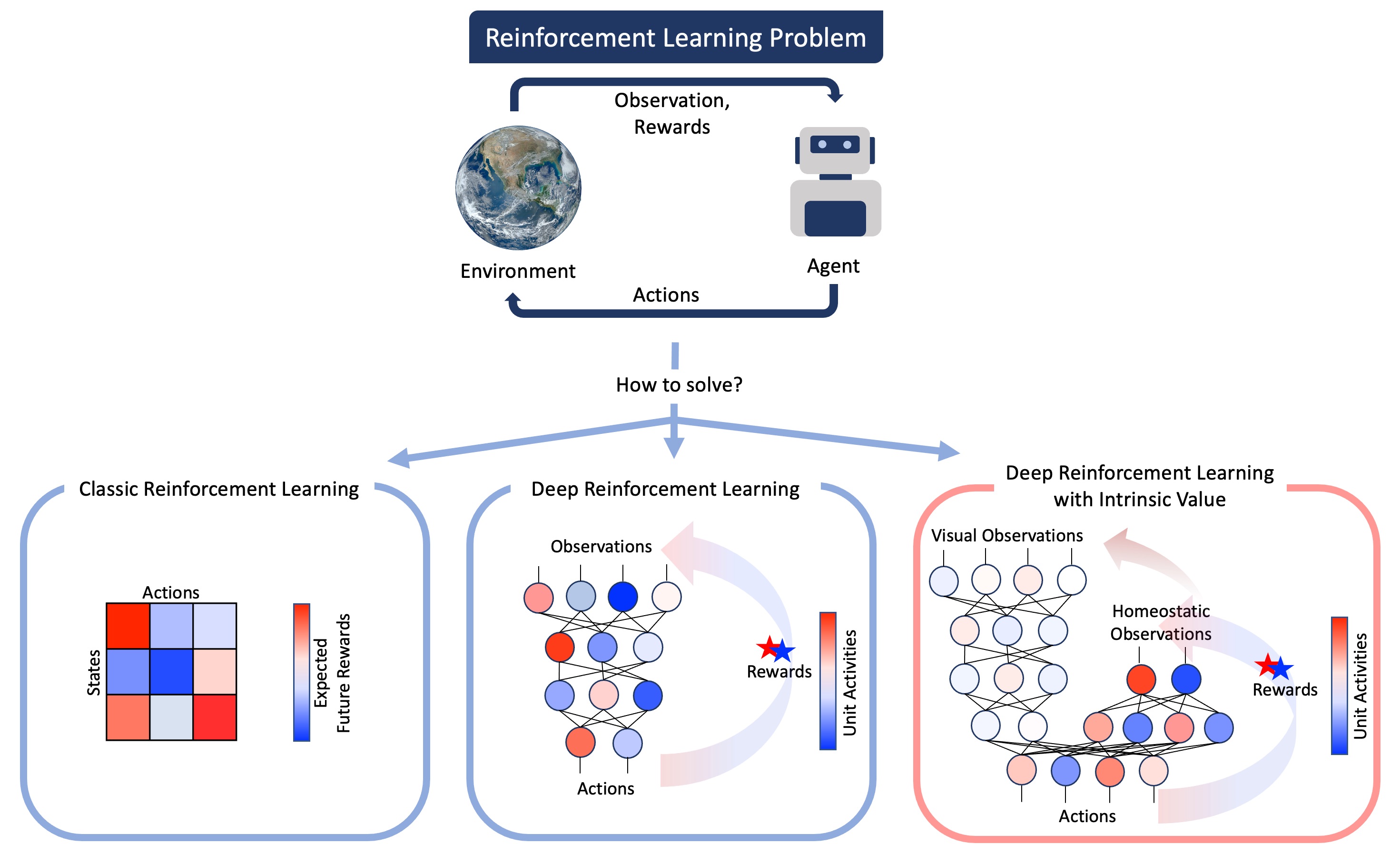
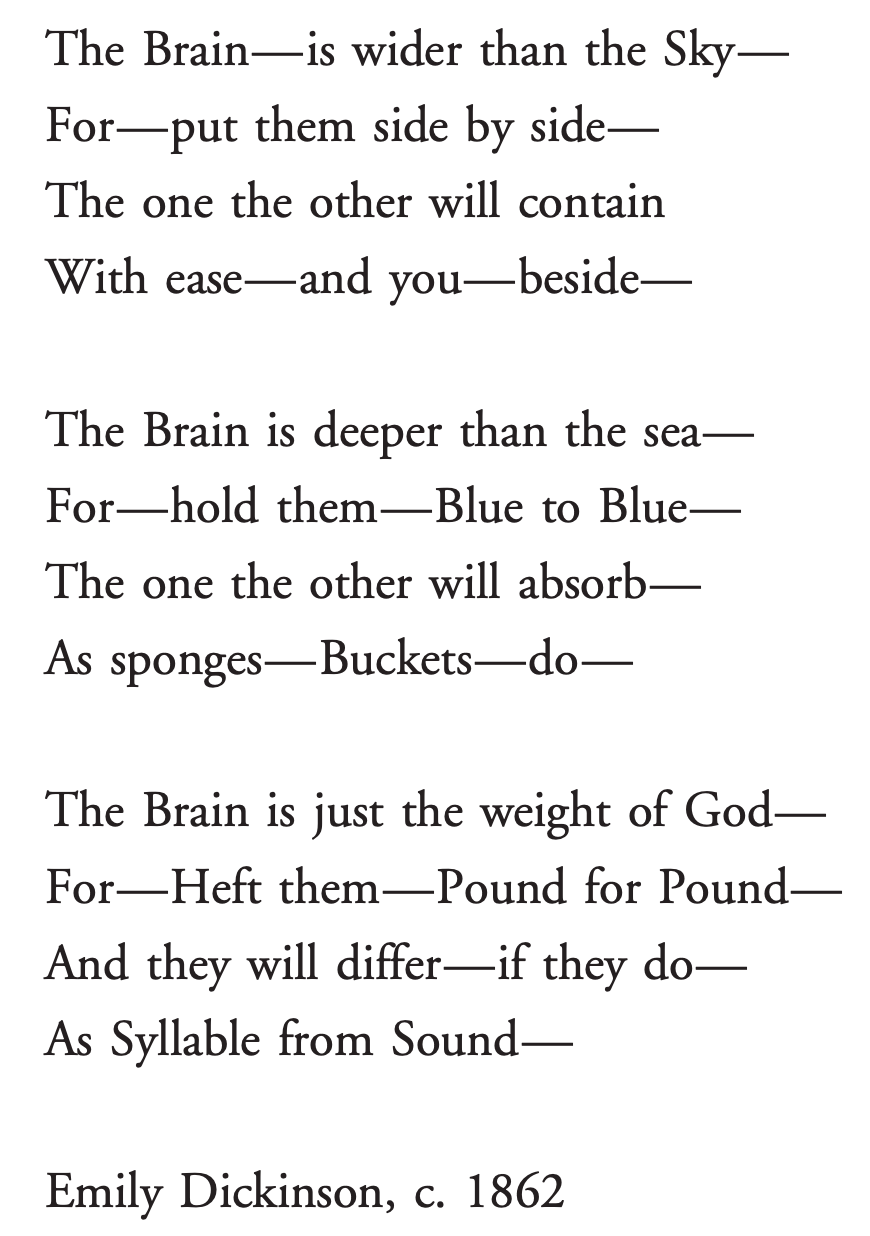